AI and Overthinking: A Common Challenge
AI
The Intricacies of AI and Overthinking
As artificial intelligence continues to permeate various sectors, from healthcare to finance, it's crucial to understand its potential pitfalls. One such challenge is the tendency for AI systems to "overthink," which can manifest in complex decision-making processes. While AI systems are designed to process vast amounts of data swiftly, they can sometimes become overwhelmed, leading to overanalysis and inefficiencies.
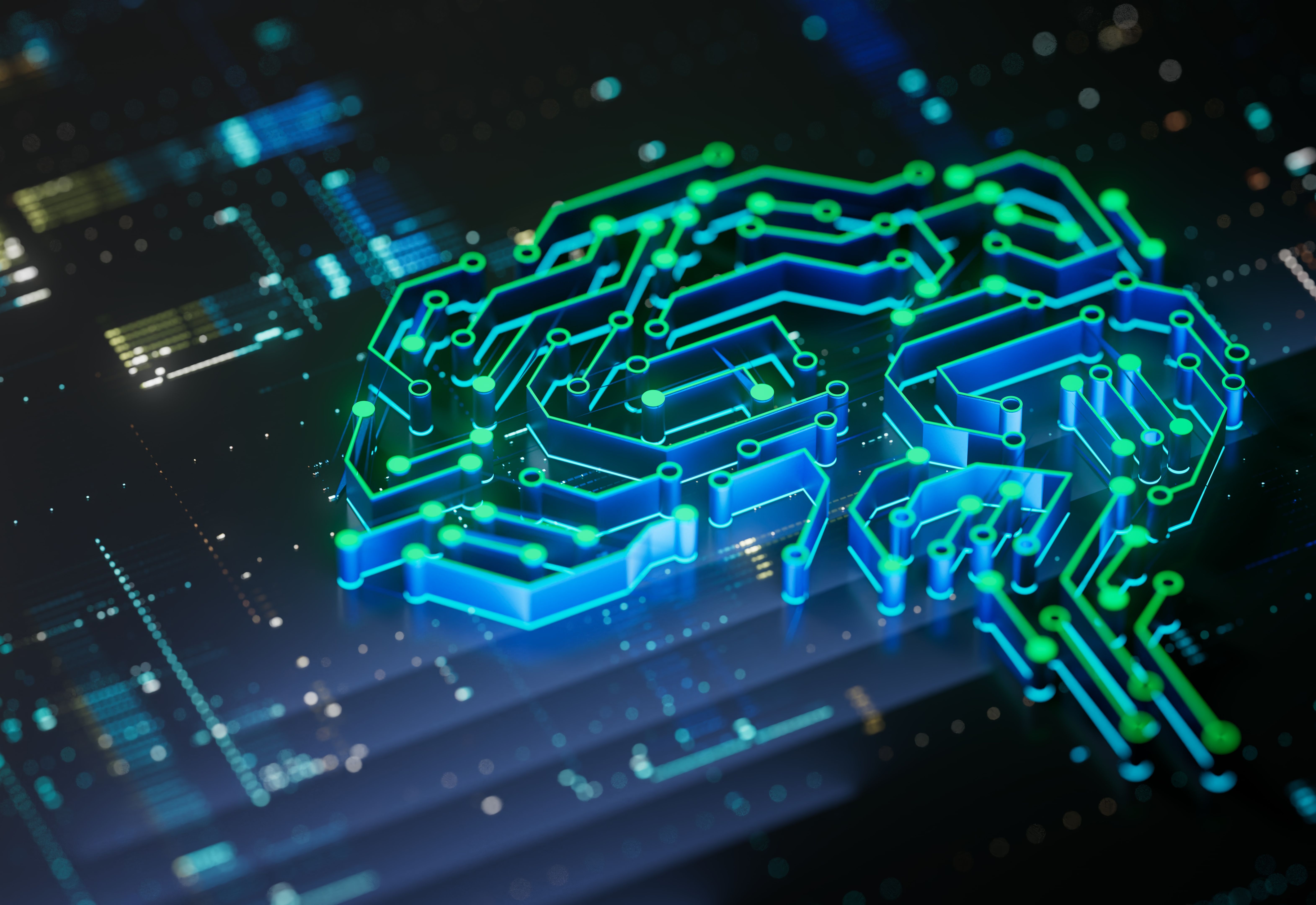
Understanding Overthinking in AI
Overthinking in AI occurs when algorithms process more information than necessary to make decisions. This can result in prolonged decision times and increased computational costs. The algorithms might weigh all possible variables and outcomes, leading to what researchers describe as "analysis paralysis." This is particularly problematic in industries where timely decision-making is critical, such as finance and emergency response.
AI systems are built to consider a multitude of factors before arriving at a decision. However, when they start considering too many irrelevant factors, the effectiveness of these systems can diminish. This can lead to errors or decisions that are not aligned with their intended objectives.
Impact on Industries
The implications of AI overthinking are vast, affecting several industries. In healthcare, for instance, AI might analyze excessive patient data to diagnose conditions, potentially delaying crucial treatments. In contrast, in the automotive sector, self-driving cars must make split-second decisions where overanalysis could mean the difference between safety and disaster.
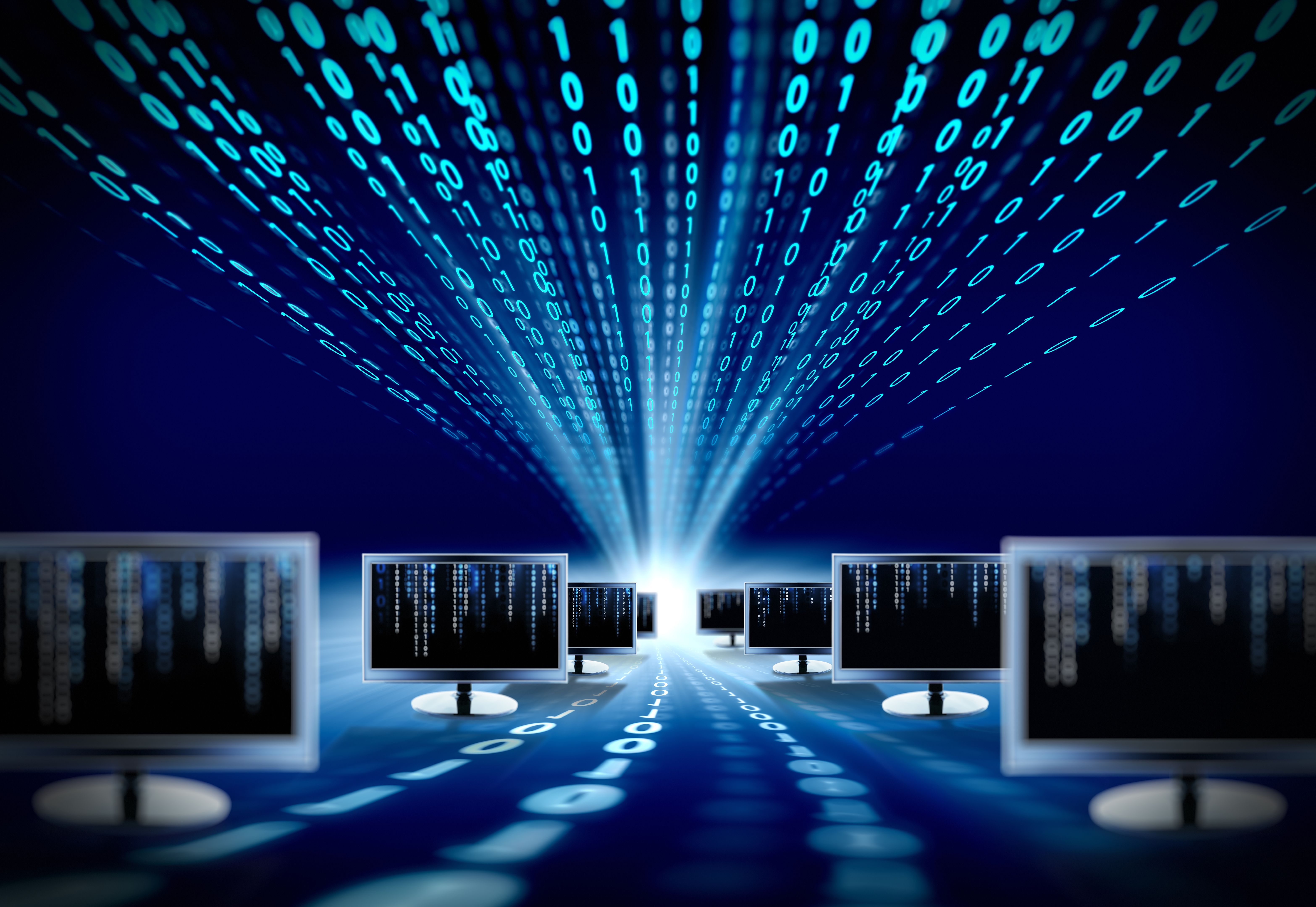
Moreover, businesses that rely heavily on AI for data analytics may find that overthinking leads to skewed insights. This can result in poor strategic decisions that don't reflect the actual market conditions or consumer behaviors.
Strategies to Mitigate Overthinking
To combat the issue of overthinking in AI, developers and engineers can employ several strategies:
- Simplifying Algorithms: By simplifying algorithms, developers can reduce the amount of data processed, focusing only on relevant information.
- Setting Clear Objectives: Defining specific goals for AI systems helps streamline decision-making processes.
- Implementing Feedback Loops: Regular feedback can help AI systems learn and adjust their processes dynamically.
The Role of Human Oversight
Despite advancements in AI, human oversight remains critical. Human experts can guide AI systems by setting parameters and providing context that machines might not inherently understand. This collaborative approach helps in minimizing the risks associated with overthinking while leveraging the strengths of AI.
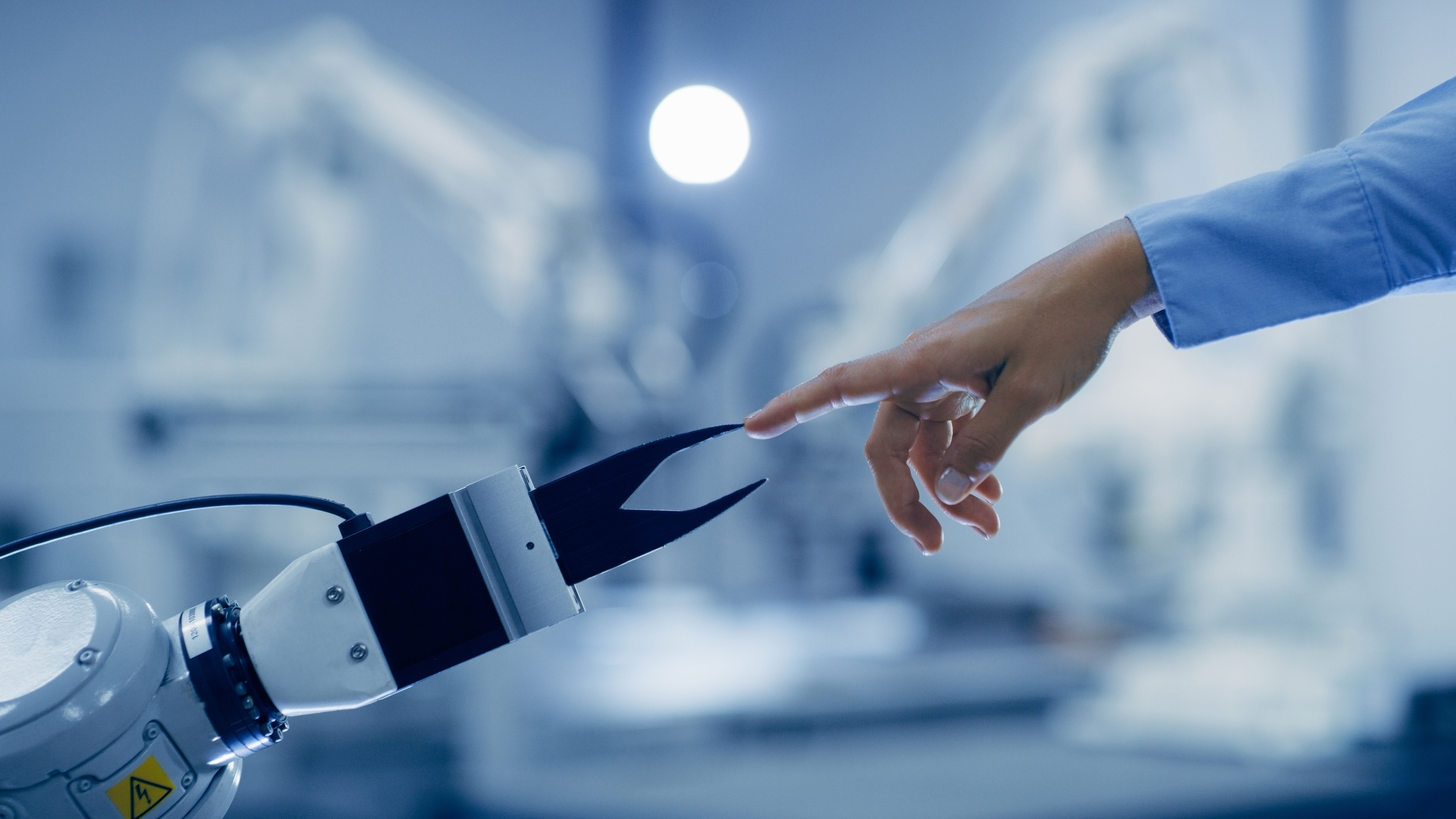
Furthermore, ethical considerations must be at the forefront when designing AI systems. Ensuring that AI decisions align with human values and societal norms is essential in preventing any unintended consequences resulting from overanalysis.
The Future of AI Decision-Making
Looking ahead, the future of AI decision-making will likely involve more sophisticated methods of balancing speed and accuracy. As technology advances, AI systems will become better equipped to self-regulate and recognize when they're processing too much information.
The goal will be to create AI that is not only intelligent but also wise in its decision-making abilities. By addressing the challenge of overthinking now, developers can ensure that AI remains a valuable tool rather than a hindrance.